Abstract
Background: The KwaZulu-Natal Sandstone Sourveld (KZN SS) is a grassland ecosystem categorised as endangered by the terms of the National Environmental Management: Biodiversity Act (Act 10 of 2004). Pressure from urbanisation has led to the remaining areas of the KZN SS being physically fragmented, causing low connectivity levels which have diminished the biological persistence of this ecosystem.
Objectives: This study aimed to quantify the overall level of habitat fragmentation of the KZN SS and determine the level of connectivity within the ecosystem, and patches of the KZN SS occurring in the eThekwini Municipal area. Using graph theory, we compared the effectiveness of broad-scale and fine-scale data sets in quantifying habitat fragmentation.
Methods: The Conefor Sensinode software, which employs the bases of graph theory, was chosen to aid in assessing fragmentation levels. The integral index of connectivity (IIC) (values range from 0 to 1, with 1 highlighting optimal connectivity) was chosen as the best index to determine landscape connectivity.
Results: The KZN SS was shown to be highly fragmented, especially at dispersal distances less than 500 m. This resulted in very low connectivity levels, with the highest IIC value recorded at 0.0063. Moreover, the use of either fine-scale or broad-scale data to measure connectivity differed substantially at 1000 m.
Conclusion: Broad-scale data were shown to overestimate habitat fragmentation and underestimate landscape connectivity. A more apt description of KZN SS connectivity levels was realised with fine-scale data. This study recommends that crucial KZN SS patches be prioritised to safeguard this endangered ecosystem.
Introduction
Human activities such as urbanisation and agriculture are the predominant drivers behind degradation and habitat loss (Ferrari, Lookingbill & Neel 2007; Hanski 1999; Schooley & Wiens 2003), which in turn threatens biological diversity worldwide (Fahrig 2001; Hanski 1999; Lindenmayer & Hobbs 2008; Schooley & Wiens 2003). Grasslands are amongst the most threatened habitats as a result of total habitat loss and degree of fragmentation (Tarboton 1997). Grasslands provide vital ecosystem services that benefit humans both directly (the provision of traditional medicinal plants and grazing for livestock) (Dzerefos & Witkowski 2001; Egoh et al. 2011, 2016) and indirectly (the sequestration of carbon for climate regulation) (Egoh et al. 2011, 2016). Additionally, South African grasslands host a high diversity of endemic flora and fauna species with 349 endemic Red List taxa of concern (South African National Biodiversity Institute [SANBI] 2015) of which 180 are threatened with extinction (SANBI 2015). South African grasslands have been identified as endangered and in need of urgent conservation (Cilliers, Muller & Drewes 2004). The KwaZulu-Natal Sandstone Sourveld (KZN SS) ecosystem is currently classified as endangered (Mucina & Rutherford 2006:512) and is distributed entirely within the sub-escarpment of KwaZulu-Natal (Figure 1).
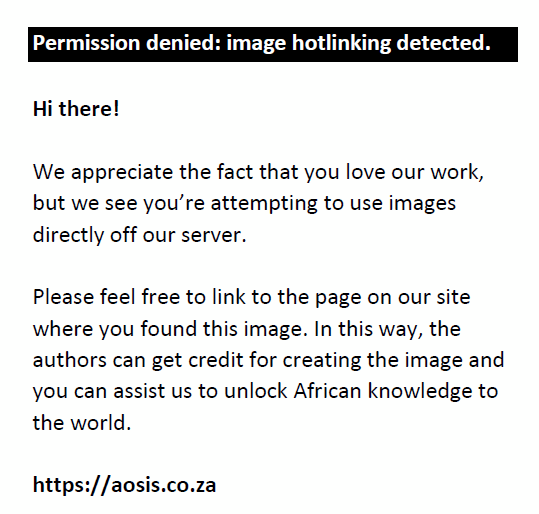 |
FIGURE 1: Original extent of the KwaZulu-Natal Sandstone Sourveld in and outside the eThekwini Metropolitan, based on the 2006 SANBI vegetation map. |
|
The connectivity of a landscape is vital for the persistence of species faced with habitat loss and fragmentation (Urban & Keitt 2001). Connectivity is often categorised into two aspects, namely structural connectivity and functional connectivity (Ferrari et al. 2007). Structural connectivity (related to the spatial structure of the landscape) is often easier to measure than functional connectivity (associated with species interactions), as it can be calculated using landscape metrics (Hanski 1999; Minor & Urban 2008; Rayfield, Fortin & Fall 2011; Urban & Keitt 2001). The management of landscape connectivity is a fundamental concern in ecology and biodiversity conservation (Minor & Urban 2008). This has increased the demand for user-driven tools that integrate connectivity within landscape planning (Fahrig 2001; Lindenmayer & Hobbs 2008; Miller 2007; Minor & Urban 2008; Visconti & Elkin 2009). The Conefor Sensinode software offers decision-makers a platform in which to quantify the importance of habitat patches for maintaining and improving functional landscape connectivity (Pascual-Hortal & Saura 2006; Pascual-Hortal & Saura 2007). This can assist decision-making in landscape planning and habitat conservation, as the measurement of landscape connectivity requires an approach that emphasises the persistence of both species and ecosystems (Fahrig 2005; Ferrari et al. 2007; Pascual-Hortal & Saura 2006; Rayfield et al. 2011; Visconti & Elkin 2009).
The KZN SS is an interesting and unique vegetation type with a naturally patchy occurrence (Mucina & Rutherford 2006). Presently, the KZN SS is classified as endangered with a small percentage remaining within an urban matrix. Habitat fragmentation has not formally been taken into account for the listing of this threatened ecosystem (Department of Environmental Affairs 2009). This study quantifies the level of habitat fragmentation of the KZN SS utilising measures of structural and functional connectivity, and assesses the influence of fine-scale and broad-scale land cover and vegetation data on habitat fragmentation measurements.
Research method and design
Study site
The KZN SS is an ecosystem currently classified as endangered on a national scale; however, provincially it is considered critically endangered (Mucina & Rutherford 2006). The KZN SS is a short, species-rich grassland with scattered low shrubs and geoxylic suffrutices. The grass and tree proportions vary and depend on many factors, especially fire. Proteaceae trees and shrubs such as Protea, Leucospermum and Faurea are common locally, and can be found on flat, sometimes rolling plateau tops and steep mountains (Mucina & Rutherford 2006). There are 12 endemic taxon species unique to the KZN SS, including Helichrysum woodii, Brachystelma modestum, Cynorkis compacta and Hesperantha gracilis (Mucina & Rutherford 2006). According to Mucina and Rutherford (2006), the altitude of the KZN SS ranges from 500 to 1100 m, but patches are found at lower altitudes (Scott-Shaw & Escott 2011). Although there is some controversy over the classification of this vegetation type, the KZN SS is considered part of the South African grasslands biome (Mucina & Rutherford 2006). The KZN SS experiences mostly summer rainfall, and receives between 700 and 1200 mm of annual rainfall (Mucina & Rutherford 2006). It occurs on the Ordovician Natal Group sandstones, upon which are skeletal, sandy soils that are nutrient-poor (Mucina & Rutherford 2006).
The fauna that inhabit the KZN SS is not very well documented (Sandy Willows-Munro [School of Life Sciences, University of KwaZulu-Natal], pers. comm., 20 October 2014). The KZN SS shares a number of endemic species with the Pondoland-Ugu Sandstone Coastal Sourveld (Mucina & Rutherford 2006). In essence, the KZN SS forms a transitional boundary between the Moist Coast Hinterland Ngongoni veld and the Moist Midlands Mist-belt grassland. Traditionally, the KZN Mist-belt grasslands support many grass-eating herbivores such as zebra, oribi and other antelope species, and provides a habitat for bird locusts (Ornithacris cyanea) (Bazelet & Samways 2014) and yellow-throated warblers (Phylloscopus ruficapillus) (Wethered & Lawes 2003). Furthermore, the KZN Mist-belt grasslands provide a feeding ground for the crowned eagles (Stephanoaetus coronatus) and raptors that hunt the numerous rodents that reside within (McPherson, Brown & Downs 2016). Furthermore, other species such as blue swallows (Hirundo atrocaerulea), the mist-belt chirping frog (Anhydrophryne ngongoniensis), and the long-toed tree frog (Leptopelis xenodactylus) are known to occur within the KZN Mist-belt grasslands (Kotze & Samways 1999; Lipsey & Hockey 2010; Wakelin & Hill 2007).
The KZN SS is found both within and outside of the eThekwini Metropolitan (hereafter referred to as EM) area (Figure 1). It originally occupied 179 671 ha (Boon et al. in press; Jewitt 2011), of which 66 611 ha is located within the EM (Boon et al. in press). The KZN SS is naturally fragmented and originally comprised three predominate areas prior to any land transformation. The extant patches of KZN SS cover roughly 2% of the EM, with a large percentage of this ecosystem type converted through agriculture and infrastructure development (Mucina & Rutherford 2006). This is principally because it is a prime agricultural area for timber and sugar cane plantations. These human activities within the EM have led to the destruction and degradation of the KZN SS, resulting in the physical fragmentation of the ecosystem.
Of the 9805 ha of KZN SS that remain within the EM, 993 ha are degraded (Boon et al. in press). Apart from land-use transformation, the extant patches of KZN SS have been degraded through the altering of fire regimes, harvesting of plants for traditional use, and nutrient enrichment from neighbouring land-uses. This has resulted in much of the grassland having been replaced by woody vegetation (Boon et al. in press).
Data and pre-processing
Assessing habitat fragmentation requires up-to-date information on the spatial extent of habitat loss. This is usually derived from land cover maps. The spatial resolution of land cover and vegetation maps influence the quantification of habitat transformation. The broad-scale data used in the analysis consisted of a 2008 land cover map (overall accuracy of 79%) (Ezemvelo KZN Wildlife, GeoTerraImage 2008) and a 2006 vegetation map (South African National Biodiversity Institute 2006). The 2008 land cover map was developed using SPOT 5 imagery (Ezemvelo KZN Wildlife, GeoTerraImage 2008; Jewitt et al. 2015). The 2006 vegetation map was developed using multiple sources such as ancillary vegetation maps, imagery (SPOT 5 2006 and 2006 aerial imagery), topographical maps and expert knowledge (Scott-Shaw & Escott 2011; South African National Biodiversity Institute 2006). The land cover and vegetation maps were combined to ascertain the level of habitat loss. The broad-scale land cover data had been classified into either ‘natural’ (predominantly made of indigenous plant species in varying degrees of ecological states as a result of soil erosion, invasive plant species and overgrazing) or ‘transformed’ (conversion of natural habitats to cultivated or urban areas where indigenous plant cover is no longer dominant). This did not differentiate habitat degradation and offered very little information pertaining to the ecological condition of the KZN SS. The fine-scale data used in the study comprised a 2011 land cover map (overall accuracy of 83.5%) (Ezemvelo KZN Wildlife, GeoTerraImage 2011; Jewitt et al. 2015) and a 2011 vegetation map (Scott-Shaw & Escott 2011). The fine-scale data used in the study offered more information regarding the ecological state on the KZN SS. The fine-scale data categorised the KZN SS into either ‘pristine’, ‘intermediate’ or ‘degraded’ based on expert knowledge and site visit (Scott-Shaw & Escott 2011).
Quantifying habitat fragmentation
Many of the metrics developed for determining connectivity only address structural connectivity or the physical connections amongst patches of a particular habitat type (Ferrari et al. 2007; Lindenmayer & Fischer 2008; Miller 2007; Minor & Urban 2008). The use of graph theory provides a more flexible set of metrics (Ferrari et al. 2007; Lindenmayer & Fischer 2008; Miller 2007; Minor & Urban 2008). The flexibility of graph theory stems from the fact that edge lengths can be defined in any way, not just by Euclidian distance between patches (Lindenmayer & Fischer 2008; Pascual-Hortal & Saura 2006; Rayfield et al. 2011; Visconti & Elkin 2009). Graph-based metrics can therefore measure functional connectivity, which accounts for species-specific habitat preferences and movement behaviours. Graph theory and spatially explicit metapopulation models that combine the physical attributes of the landscape with limited species information can be used to provide a measure of potential connectivity (Ferrari et al. 2007; Lindenmayer & Fischer 2008; Miller 2007; Minor & Urban 2008).
Conefor Sensinode has been suggested to have the greatest benefit for conservation problems regarding landscape connectivity as it includes new connectivity metrics based on the habitat availability concept (Pascual-Hortal & Saura 2006, 2007). The habitat availability concept enables a patch to be considered as a space where connectivity occurs. This enables habitat patch area and the connections between different patches to be integrated into a single measure (Pascual-Hortal & Saura 2006).
The Conefor Sensinode software has several connectivity indices available for use, of which the integral index of connectivity (IIC) (Pascual-Hortal & Saura 2006) and the probability of connectivity (PC) are the most highly recommended indices (Pascual-Hortal & Saura 2007). The software considers connectivity from a functional point of view. Hence, not only is the spatial configuration of the landscape (structural connectivity) taken into account, but also it encompasses the dispersal distances of species and their interaction with the physical structure of the landscape (functional connectivity) in the analysis (Pascual-Hortal & Saura 2007; Theobald 2006; Tischendorf & Fahrig 2000). The required inputs for the software are, therefore, both the spatial structure of the landscape and the dispersal distance of the study species.
Analysis
Landscape connectivity is species-specific and different species have diverse levels of dispersal and movement. Dispersal data on key KZN SS species were not available so a range of different dispersal distances (50, 100, 250, 500, and 1000 m) were taken into consideration for the study. This enabled the representation of a wide range of dispersal distances for grassland flora and fauna. The 50 m dispersal distance represents the dispersal range of smaller mammals such as grassland mice (Kollmann & Schill 1996) and the autochorous dispersal of seeds in plants (Higgins, Nathan & Cain 2003), whilst the 1000 m distance is more representative of medium-sized mammals such as duiker (Lawes, Mealin & Piper 2000). The range of dispersal distances used can be applied to many species, but the results produced may not necessarily reflect the movement patterns of all the organisms in the landscape. The study primarily focused on the 250 m dispersal distance to indicate the impact of habitat fragmentation at an intermediate dispersal range, as preliminary analysis using different distances exhibited minimal changes from one distance to the next. The attributes of the four data sets used in the analysis – namely broad-scale KZN SS (full extent); broad-scale KZN SS (EM only); fine-scale KZN SS in good condition (EM only); and fine-scale KZN SS in any condition (EM only) – are summarised in Table 1. The study did not consider species-specific data as our knowledge of the KZN SS is limited and fauna that inhabit the KZN SS are not well documented.
TABLE 1: Summary of data used for analysis, with variation of resolution, geographical extent and ecological condition. |
Determining the connectivity of different fragments
Connectivity can be quantified as either binary or probabilistic. Binary considers each habitat patch as either connected or unconnected and does not factor in the strength of the connection between patches. Probabilistic, however, considers the movement of fauna as a means to estimate the strength of the connection between habitat patches (Pascual-Hortal & Saura 2007). There are nine different types of binary indices available for use through the Conefor software. The integral index of connectivity (IIC) was chosen as the best binary index for calculating landscape connectivity measurements (Pascual-Hortal & Saura 2007). The advantage of the IIC lies in the formula, which allows for patch quality and connectivity to be integrated, allowing each habitat patch to be considered as an area where connectivity takes place (Pascual-Hortal & Saura 2007). The IIC is calculated by the formula indicated below, with the output values ranging from 0 to 1, with 1 highlighting optimal connectivity.
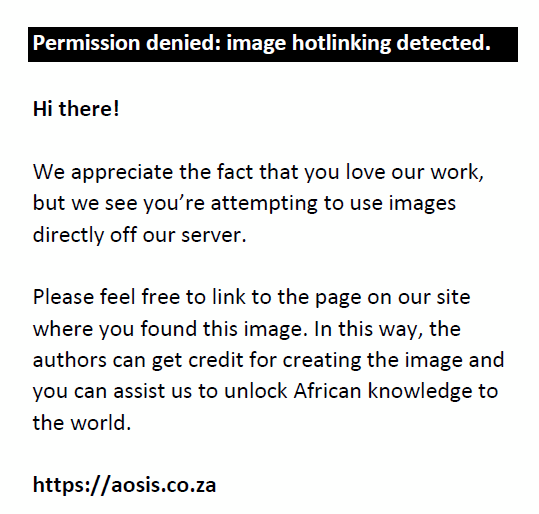
Where: n is the total number of nodes (the habitat area considered for the connectivity analysis) (Pascual-Hortal & Saura 2006), ai and aj are the attributes (e.g. habitat area) of nodes i and j, nlij is the number of links (the connection between habitat nodes in the landscape) in the shortest path between patches i and j, and AL is the maximum landscape attribute (the total landscape area, consisting of both habitat and non-habitat areas) (Pascual-Hortal & Saura 2006, 2007).
The individual patches were arranged and prioritised according to their overall contribution to the connectivity of the landscape. Larger and less fragmented patches were viewed as better connected, with fewer components (a set of nodes which contain pathways between every pair of nodes) representing a more connected landscape (Pascual-Hortal & Saura 2007).
Quantifying overall landscape connectivity
In order to determine the extent of the overall landscape connectivity of the KZN SS, two connectivity indices were used, namely the number of components and the IIC. These specific connectivity indices were chosen as they do not demonstrate the same problems associated with many other connectivity indices. For instance, where there is an increase in connectivity with increased fragmentation, or no connectivity predicted for a landscape occupied by one large habitat patch, or a lack of response of the index to the loss of a large isolated patch (Lindenmayer & Fischer 2008; Pascual-Hortal & Saura 2007; Visconti & Elkin 2009). As connectivity across the landscape increases, the number of components will decrease. More connected landscapes will tend to consist of one large component in which all the habitat patches are connected. As the landscape becomes more connected, the percentage of the available habitat area that is in the largest component will also increase (Fahrig 2001; Lindenmayer & Hobbs 2008; Miller 2007; Visconti & Elkin 2009).
Results
The degree of habitat fragmentation
The KZN SS is highly fragmented at a small dispersal distance and as the dispersal distance increases from 500 m onwards, there is a much lower level of fragmentation. The majority of the KZN SS is located outside the EM. This explains the high number of components and greater level of fragmentation seen with the ‘broad-scale KZN SS (full extent)’ data set (Figure 2), which has an excess of 1200 components, in comparison to the other data sets (Figures 2 and 3), which have less than 200 components. The number of components is considerably less when fine-scale data are used. Furthermore, the number of components can be observed to have changed substantially when small dispersal distances are used for fine-scale data; however, with the use of large distances it can be noted that fine-scale data do not show such a major difference. The slight variation between ‘fine-scale KZN SS in good condition (EM only)’ and ‘fine-scale KZN SS in any condition (EM only)’ in Figure 3 implies that the inclusion of patches regardless of their ecological condition does not have a substantial impact upon the level of fragmentation experienced.
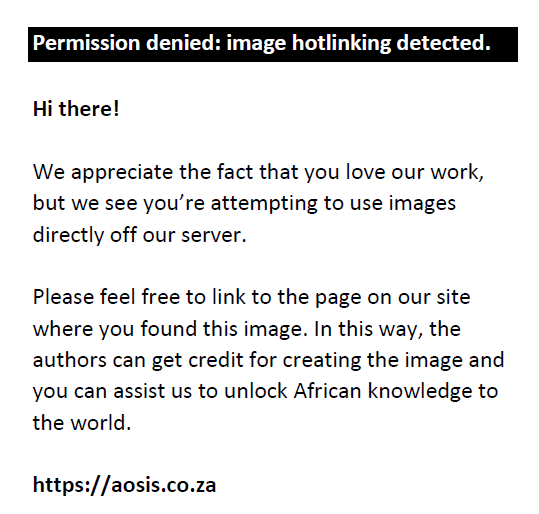 |
FIGURE 2: The change in number of components in relation to dispersal distance (the greater the number of components, the higher the level of fragmentation) for broad-scale KZN SS (full extent) and broad-scale KZN SS (EM only). |
|
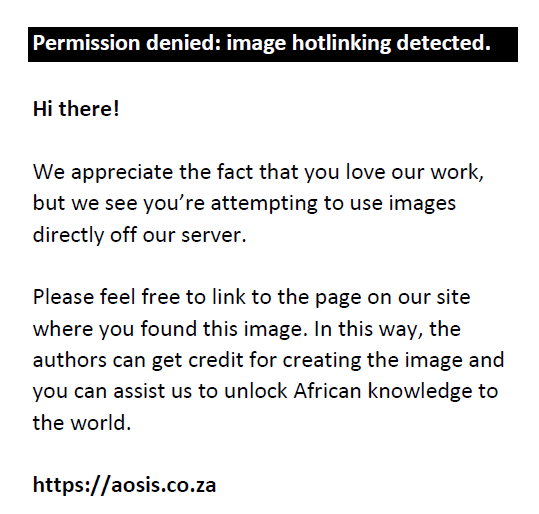 |
FIGURE 3: The change in number of components experienced in relation to dispersal distance (the greater the number of components, the higher the level of fragmentation) for broad-scale KZN SS (EM only), fine-scale KZN SS in a good condition (EM only), and fine-scale KZN SS in any condition (EM only). |
|
Connectivity levels
The overall landscape connectivity of the KZN SS for each of the different data sets is depicted in Figure 4. The general trend displayed for each data set is an increase in connectivity as the dispersal distance increases. The inclusion or exclusion of degraded patches had an important impact upon the overall landscape connectivity. The ‘fine-scale KZN SS in any condition (EM only)’ data set (Figure 4), which included degraded patches, showed a considerably higher level of connectivity. This outcome was expected and it indicates that it is possible for degraded patches to be stepping-stones between more desirable habitats. In addition, degraded patches are particularly important for species with a dispersal distance in excess of 500 m, evident by the higher IIC. There is an important difference between the uses of either broad-scale or fine-scale data evident in Figure 4. At 1000 m, both broad-scale and fine-scale data that dealt with patches within the EM have a substantial difference of 85% in their connectivity levels. The broad-scale data set only captured large patches and did not capture many of the smaller patches. The smaller patches (included in the fine-scale data) increased the level of connectivity. Broad-scale data tend to underestimate levels of connectivity. The patches located within the EM show a higher level of connectivity, possibly owing to the smaller distance covered within the municipal area as compared to the distance covered by the entire extent of the KZN SS.
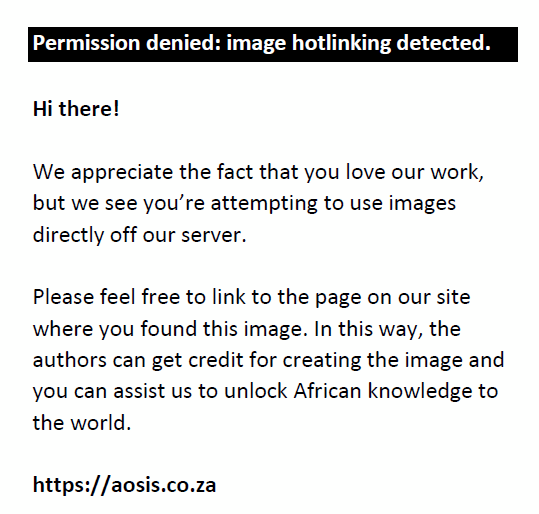 |
FIGURE 4: Overall landscape connectivity levels of the KZN SS (expressed as the change in the integral index of connectivity [IIC] with dispersal distance) using both broad-scale and fine-scale data. |
|
The individual importance of each patch concerning the level of connectivity of the landscape is depicted in Figure 5, showing the significance of each patch and therefore the loss of connectivity should this patch be lost. The regions highlighted in red are considered the most connected and hence are essential in ensuring the connectivity of the region. Most of the patches essential to connectivity are located outside the EM, with connectivity levels slightly increasing with dispersal distance. There are several patches located outside the EM that are crucial to connectivity, of which the patch located to the south-east of KoShange is the most crucial in the landscape (Figure 5). Patches located near Assagay and Crest Holme are crucial for connectivity within the EM (Figure 6A). However, when placed in the broader context of the landscape, these patches hold only a moderate level of importance for connectivity (Figure 5).
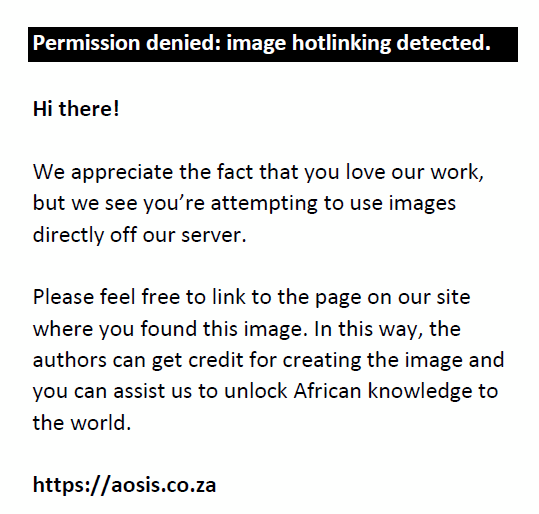 |
FIGURE 5: The importance that each patch of KZN SS in a natural condition contributes towards the connectivity (expressed as the delta integral index of connectivity (dIIC), the output values range from 0 to 1, with 1 highlighting optimal connectivity) for the entire KZN SS within the 2006 SANBI vegetation map (broad-scale KZN SS (full extent)), based on 250 m dispersal distance. |
|
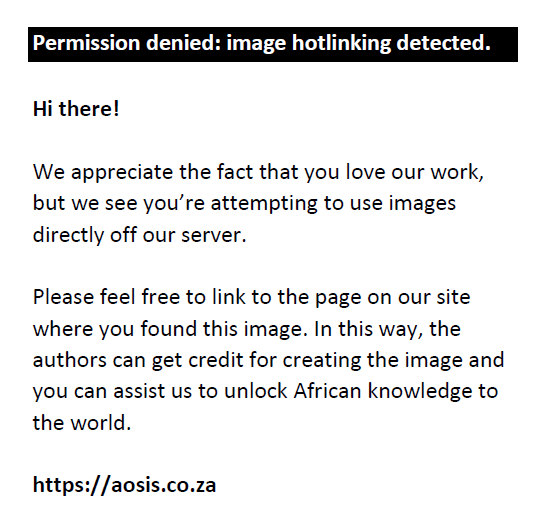 |
FIGURE 6: The importance that each patch of KZN SS contributes towards the connectivity (expressed as the delta integral index of connectivity (dIIC), the output values range from 0 to 1, with 1 highlighting optimal connectivity) of KZN SS patches that fall within the EM at a dispersal distance of 250 m, based on: (a), broad-scale KZN SS (EM only); (b) fine-scale KZN SS in good condition (EM only), and (b) fine-scale KZN SS in any condition (EM only). |
|
The majority of vital patches are located away from the N3, as roads have the ability to act either as conduits or as a barrier, and in so doing, inhibiting movement of mammals and reptiles. The inclusion of degraded patches (fine-scale KZN SS in any condition (EM only)) has shown to increase the connectivity levels of a number of different patches within the landscape, which is apparent upon examination of the patches located at Sterkspruit, Vutha, Olwambeni and Crest Holme (Figure 6B and 6C). These patches increase in connectivity with the inclusion of degraded patches regardless of dispersal distance. There are noticeable differences between the uses of broad-scale and fine-scale data, which is discernible between Figure 6A (broad-scale KZN SS (EM only)) and Figure 6B (fine-scale KZN SS in good condition (EM only)). One can see that the patches of KZN SS located at Assagay and Crest Holme have been broken down into smaller fragments not holding as much importance as they did in Figure 6A (broad-scale KZN SS (EM only)). In addition, the group of fragments located near Sterkspruit (fine-scale KZN SS in good condition (EM only)), which held a relatively high importance, is absent (Figure 6A and 6B).
Discussion
The results indicate that, compared to its naturally occurring state, the KwaZulu-Natal Sandstone Sourveld is heavily fragmented. This could have severe implications for ecosystem functioning, especially for species with shorter dispersal distances (Cushman 2006; Whitcomb et al. 1981). These species may not be able to move freely if patches of KZN SS are not well connected, leading to local species extinction within isolated patches (e.g. Trenham 2001). Taxa which have a small dispersal radius – e.g. arachnids (Bonte et al. 2003), juvenile amphibians (Trenham 2001), invertebrates (Kotze & Samways 1999) and rodents (Kollmann & Schill 1996) – are more likely to be vulnerable to this high level of fragmentation. Species with larger dispersal distances (usually predators) are less likely to be affected by fragmentation (LaRue & Nielsen 2008; Wells et al. 2008). Future studies on habitat fragmentation and connectivity would benefit from species-specific data to incorporate movement patterns of species.
Well-connected fragments can form a corridor to facilitate the movement of species between the different habitat fragments (Bennett, Henein & Merriam 1994; Doko et al. 2011; LaRue & Nielsen 2008; Urban & Keitt 2001) and enable species persistence. However, the study ascertained that the KZN SS is not well connected – the highest IIC value amongst the data sets was recorded at 0.0063 (fine-scale KZN SS in any condition (EM only)) (Figure 4). This IIC value is considerably low in comparison to data obtained by Fourie, Rouget and Lotter (2015) for the Mpumalanga grasslands, where the lowest IIC value recorded was 0.049 for a 1000 m dispersal distance. The low connectivity level for the KZN SS is directly linked with the high degree of habitat loss and fragmentation currently being experienced. A similar study by Garcia-Feced, Saura and Elena-Rossello (2011) yielded minimum IIC values of 0.45 for forests in the Mediterranean region.
Spatial resolution of the land cover and, to some extent, habitat condition influenced the quantification of habitat fragmentation and connectivity. A comparison between the broad-scale data for both in and outside the EM showed that the habitat patches outside the EM had a higher level of connectivity. The proposed approach can be used to identify key patches (such as the patch located at Hoqweni (Figure 5)) to maintain habitat connectivity in the landscape. Degraded patches improved connectivity levels, providing stepping stones for species with smaller dispersal distances to gain access to pristine patches. Fourie et al. (2015) concluded that degraded patches are important for the connectivity of grasslands in Mpumalanga. This study also suggests that key degraded patches, if rehabilitated, would improve the connectivity of the landscape.
Broad-scale data over-estimated the level of habitat fragmentation, which is counter-intuitive to other studies (e.g. Payet et al. 2013). Thompson et al. (2009) showed that the use of broad-scale data could underestimate the quantification of degraded habitat. Map misclassification, which can result in errors with landscape pattern indices (such as mean patch size, patch shape and clumpiness), could have influenced the level of habitat fragmentation experienced with broad-scale data (Langford et al. 2006). The use of fine-scale data showed a higher IIC, possibly as a result of more stepping stone patches, and displayed a higher level of connectivity at smaller distances. The fine-scale data better depicted the current state of the KZN SS. High spatial resolution data are vital to adequately monitor and delineate important fragments (Boyle et al. 2014).
The proposed approach enabled the identification of essential patches to maintain habitat connectivity of this threatened ecosystem. Such information can inform local planning and management in different ways. Critical patches of KZN SS (and other ecosystems) have been included in the Durban Metropolitan Open Space System (DMOSS) framework albeit based on a different approach (Boon et al. in press). The DMOSS, incorporated in eThekwini’s town planning schemes, is a critical instrument to ensure that the development planning and assessment process adequately consider biodiversity concerns (Roberts et al. 2012). Moreover, important patches of KZN SS are conserved through land acquisitions or agreements with landowners. The Hill ‘n Dale Estate is a prime example of the eThekwini Municipality’s purchase of private property and conversion into a nature reserve that protects important patches of KZN SS. Restoration efforts conducted by eThekwini Municipality, such as alien plant clearing (through the Working for Ecosystems project) and fire management (through the Working on Fire programme), can be directed towards critical patches of KZN SS. Lastly, we recommend that critical patches of KZN SS (and other threatened ecosystems) be included in the Durban climate change strategy.
Acknowledgments
This study was supported by eThekwini Municipality through the Durban Research Action Partnership: KwaZulu-Natal Sandstone Sourveld Programme. The South African Research Chairs Initiative of the Department of Science and Technology and the National Research Foundation of South Africa (grant no 84157) financially supported the research.
Competing interests
The authors declare that they have no financial or personal relationships which may have inappropriately influenced them in writing this article.
Authors’ contributions
R.N. was enrolled as an MSc student at the University of KwaZulu-Natal during the time the research was undertaken. He conceptualised the study, performed all analyses and led the writing of the manuscript under the guidance and supervision of M.R. and O.M.
References
Bazelet, C.S. & Samways, M.J., 2014, ‘Habitat quality of grassland fragments affects dispersal ability of a mobile grasshopper, Ornithacris cyanea (Orthoptera: Acrididae)’, African Entomology 22(4), 714–725. http://dx.doi.org/10.4001/003.022.0405
Bennett, A.F., Henein, K. & Merriam, G., 1994, ‘Corridor use and the elements of corridor quality: Chipmunks and fencerows in a farmland mosaic’, Biological Conservation 68, 155–165. http://dx.doi.org/10.1016/0006-3207(94)90347-6
Bonte, D., Lens, L., Maelfait, J.P., Hoffmann, M. & Kuijken, E., 2003, ‘Patch quality and connectivity influence spatial dynamics in a dune wolfspider’, Population Ecology 135, 227–233. http://dx.doi.org/10.1007/s00442-003-1195-6
Boon, R., Cockburn, J., Douwes, E., Govender, N., Ground, L., Mclean, C. et al., 2016, ‘Managing a threatened ecosystem in an urban biodiversity hotspot: Durban, South Africa’ Bothalia 46(2), a2112. http://dx.doi.org/10.4102/abc.v46i2.2112
Boyle, S.A., Kennedy, C.M., Torres, J., Coleman, K., Perez-Estigarribia, P.E. & De la Sancha, N., 2014, ‘High-resolution satellite imagery is an important yet underutilized resource in conservation biology’, PLoS One 9(1), e86908. http://dx.doi.org/1.0.1371/journal.pone.0086908
Cilliers, S.S., Muller, N. & Drewes, E., 2004, ‘Overview on urban nature conservation: Situation in the western-grassland biome of South Africa’, Urban Forestry and Urban Greening 3, 49–62. http://dx.doi.org/10.1016/j.ufug.2004.04.003
Cushman, S.A., 2006, ‘Effects of habitat fragmentation on amphibians: A review and prospectus’, Biological Conservation 128, 231–240. http://dx.doi.org/10.1016/j.biocon.2005.09.031
Department of Environmental Affairs, 2009, Threatened ecosystems in South Africa: General information (notice 1477 of 2009), Government Gazette 32689, 6 November, Government Printer, Pretoria.
Doko, T., Fukui, H., Kooiman, A., Toxopeus, A.G., Ichinose, T., Chen, W. et al., 2011, ‘Identifying habitat patches and potential ecological corridors for remnant Asiatic black bear (Ursus thibetanus japonicus) populations in Japan’, Ecological Modelling 222, 748–761. http://dx.doi.org/10.1016/j.ecolmodel.2010.11.005
Dzerefos, C.M. & Witkowski, E.T.F., 2001, ‘Density and potential utilisation of medicinal grassland plants from Abe Bailey Nature Reserve, South Africa’, Biodiversity and Conservation 10, 875–896. http://dx.doi.org/10.1023/A:1013177628331
Egoh, B.N., Bengtsson, J., Lindborg, R., Bullock, J.M., Dixon, A.P. & Rouget, M., 2016, ‘The importance of grasslands in providing ecosystem services: Opportunities for poverty alleviation’, in M. Potschin, R. Haines-Young, R. Fish & R.K. Turner (eds.), Routledge handbook of ecosystem services, pp. 421–441, Taylor & Francis, Abingdon.
Egoh, B.N., Reyers, B., Rouget, M. & Richardson, D.M., 2011, ‘Identifying priority areas for ecosystem service management in South African grasslands’, Journal of Environment Management 92(6), 1642–1650. http://dx.doi.org/10.1016/j.jenvman.2011.01.019
Ezemvelo KZN Wildlife, GeoTerraImage, 2008, 2008 KZN Province land-cover mapping (from SPOT5 satellite imagery circa 2008): Data users report and metadata (version 1), Ezemvelo KZN Wildlife, Pietermaritzburg; 2010 [unpublished report].
Ezemvelo KZN Wildlife, GeoTerraImage, 2011, 2011 KZN Province land-cover mapping (from SPOT5 satellite imagery circa 2011): Data users report and metadata (version 1d)’ Ezemvelo KZN Wildlife, Pietermaritzburg;2013 [unpublished report].
Fahrig, L., 2001, ‘How much habitat is enough?’, Biological Conservation 100, 65–74. http://dx.doi.org/10.1016/S0006-3207(00)00208-1
Fahrig, L., 2005, ‘Estimating minimum habitat for population persistence’, in D.B. Lindenmayer & R.J. Hobbs (eds.), Managing and designing landscapes for conservation: Moving from perspectives to principles, p. 64–75, Blackwell, Victoria, Australia.
Ferrari, J.R., Lookingbill, T.R. & Neel, M.C., 2007, ‘Two measures of landscape-graph connectivity: Assessment across gradients in area and configuration’, Landscape Ecology 22, 1315–1323. http://dx.doi.org/10.1007/s10980-007-9121-7
Fourie, L., Rouget, M. & Lotter, M., 2015, ‘Landscape connectivity of the grassland biome in Mpumalanga, South Africa’, Austral Ecology 40, 67–76. http://dx.doi.org/10.1111/aec.12169
Garcia-Feced, C., Saura, C. & Elena-Rossello, R., 2011, ‘Improving landscape connectivity in forest districts: A two-stage process for prioritizing agricultural patches for reforestation’, Forest Ecology and Management 261, 154–161. http://dx.doi.org/10.1016/j.foreco.2010.09.047
Hanski, I., 1999, ‘Habitat connectivity, habitat continuity, and metapopulations in dynamic landscapes’, Oikos 77, 209–219. http://dx.doi.org/10.2307/3546736
Higgins, S.I., Nathan, R. & Cain, M.L., 2003, ‘Are long-distance dispersal events in plants usually caused by nonstandard means of dispersal’, Ecology 84(8), 1945–1956. http://dx.doi.org/10.1890/01-0616
Jewitt, D.J., 2011, Conservation targets and status for vegetation types in KZN, Ezemvelo KZN Wildlife, Pietermaritzburg.
Jewitt, D.J., Goodman, P.S., Erasmus, B.F.N., O’Connor, T.G. & Witkowski, E.T.F., 2015, ‘Systematic land-cover change in KwaZulu-Natal South Africa: Implications for biodiversity’, South African Journal of Science 111(9), 1–9.
Kollmann, J. & Schill, H.P., 1996, ‘Spatial patterns of dispersal, seed predation and germination during colonization of abandoned grassland by Quercus petraea and Corylus avellana’, Vegetatio 125, 193–205. http://dx.doi.org/10.1007/BF00044651
Kotze, J. & Samways, M.J., 1999, ‘Invertebrate conservation at the interface between the grassland matrix and natural Afromontane forest fragments’, Biodiversity and Conservation 8, 1339–1363. http://dx.doi.org/10.1023/A:1008945302029
Langford, W., Gergel, S., Dietterich, T. & Cohen, W., 2006, ‘Map misclassification can cause large errors in landscape pattern indices: Examples from habitat fragmentation’, Ecosystems 9, 474–488. http://dx.doi.org/10.1007/s10021-005-0119-1
LaRue, M.A. & Nielsen, C.K., 2008, ‘Modelling potential dispersal corridors for cougars in Midwestern North America using least-cost path methods’, Ecological Modelling 212, 372–381. http://dx.doi.org/10.1016/j.ecolmodel.2007.10.036
Lawes, M.J., Mealin, P.E. & Piper, S.E., 2000, ‘Patch occupancy and potential metapopulation dynamics of three forest mammals in fragmented Afromontane Forest in South Africa’, Conservation Biology 14(4), 1088–1098. http://dx.doi.org/10.1046/j.1523-1739.2000.99120.x
Lindenmayer, D.B. & Fischer, J., 2008, ‘Landscape models for use in studies of landscape change and habitat fragmentation’, in D.B. Lindenmayer & R.J. Hobbs (eds.), Managing and designing landscapes for conservation: Moving from perspectives to principles, p. 35–41, Blackwell, Victoria, Australia.
Lindenmayer, D.B. & Hobbs, R.J., 2008, ‘Synthesis: Habitat, habitat loss and patch sizes’, in D.B. Lindenmayer & R.J. Hobbs (eds.), Managing and designing landscapes for conservation: Moving from perspectives to principles, p. 96–98, Blackwell, Victoria, Australia.
Lipsey, M.K. & Hockey, P.A.R., 2010, ‘Do ecological networks in South African commercial forests benefit grassland birds? A case study of a pine plantation in KwaZulu-Natal’, Agriculture, Ecosystems and Environment 137(1), 133–142. http://dx.doi.org/10.1016/j.agee.2010.01.013
McPherson, S.C., Brown, M. & Downs, C.T., 2016, ‘Crowned eagle nest sites in an urban landscape: Requirements of a large eagle in the Durban Metropolitan Open Space System’, Landscape and Urban Planning 146, 43–50. http://dx.doi.org/10.1016/j.landurbplan.2015.10.004
Miller, J.R., 2007, ‘Habitat and landscape design: Concepts, constraints and opportunities’, in D.B. Lindenmayer & R.J. Hobbs (eds.), Managing and designing landscapes for conservation: Moving from perspectives to principles, p. 81–89, Blackwell, Victoria, Australia.
Minor, E. & Urban, D., 2008, ‘A graph-theory framework for evaluating landscape connectivity and conservation planning’, Conservation Biology 22(2), 297–307. http://dx.doi.org/10.1111/j.1523-1739.2007.00871.x
Mucina, L. & Rutherford, M.C. (eds.), 2006, The vegetation of South Africa, Lesotho and Swaziland, South African National Biodiversity Institute, Pretoria (Strelitzia no. 19).
Pascual-Hortal, L. & Saura, S., 2006, ‘Comparison and development of new graph-based landscape connectivity indices: Towards the priorisation of habitat patches and corridors for conservation’, Landscape Ecology 21(7), 959–967. http://dx.doi.org/10.1007/s10980-006-0013-z
Pascual-Hortal, L. & Saura, S., 2007, ‘Impact of spatial scale on the identification of critical habitat patches for the maintenance of landscape connectivity’, Landscape and Urban Planning 83(2–3), 176–186. http://dx.doi.org/10.1016/j.landurbplan.2007.04.003
Payet, K., Rouget, M., Esler, K., Reyers, B., Rebelo, T., Thompson, M. et al., 2013, ‘Effect of land cover and ecosystem mapping on ecosystem-risk assessment in the Little Karoo, South Africa’, Conservation Biology 27(3), 531–541. http://dx.doi.org/10.1111/cobi.12065
Rayfield, B., Fortin, M. & Fall, A., 2011, ‘Connectivity for conservation: A framework to classify network measures’, Ecology 92(4), 847–858. http://dx.doi.org/10.1890/09-2190.1
Roberts, D., Boon, R., Diederichs, N., Douwes, E., Govender, N., Mcinness, A. et al., 2012, ‘Exploring ecosystem-based adaptation in Durban, South Africa: “Learning-by-doing” at the local government coal face’, Environment and Urbanization 24, 167–195. http://dx.doi.org/10.1177/0956247811431412
Schooley, R.L. & Wiens, J.A., 2003, ‘Finding habitat patches and directional connectivity’, Oikos 102, 559–570. http://dx.doi.org/10.1034/j.1600-0706.2003.12490.x
Scott-Shaw, C.R. & Escott, B.J. (eds.), 2011, KwaZulu-Natal provincial pre-transformation vegetation type map – 2011, Unpublished GIS Coverage [kznveg05v2_1_11_wll.zip], Biodiversity Conservation Planning Division, Ezemvelo KZN Wildlife, Cascades, Pietermaritzburg.
South African National Biodiversity Institute, 2006, Vegetation map of South Africa, Lesotho and Swaziland 2006 GIS metadata: Detailed report, South African National Biodiversity Institute, Claremount, South Africa.
South African National Biodiversity Institute (SANBI), 2015, Statistics: Red list of South African plants version 2015.1, viewed 11 August 2016, from Redlist.sanbi.org
Tarboton, W.R., 1997, ‘South Africa’s grasslands: The Cinderella biome’, Africa – Birds and Birding 2, 57–60.
Theobald, D.M., 2006, ‘Exploring the functional connectivity of landscapes using landscape networks’, in K.R. Crooks & M. Sanjayan (eds.), Connectivity conservation, pp. 416–443, Cambridge University Press, New York.
Thompson, M., Vlok, J., Rouget, M., Hoffman, M., Balmford, A. & Cowling, R., 2009, ‘Mapping grazing-induced degradation in a semi-arid environment: A rapid and cost effective approach for assessment and monitoring’, Environmental Management 43, 585–596. http://dx.doi.org/10.1007/s00267-008-9228-x
Tischendorf, L. & Fahrig, L., 2000, ‘On the usage and measurement of landscape connectivity’, Oikos 90, 7–19. http://dx.doi.org/10.1034/j.1600-0706.2000.900102.x
Trenham, P.C., 2001, ‘Terrestrial habitat use by adult California tiger salamanders’, Journal of Herpetology 35, 343–346. http://dx.doi.org/10.2307/1566130
Urban, D. & Keitt, T., 2001, ‘Landscape connectivity: A graph-theoretic perspective’, Ecology 82, 1205–1218. http://dx.doi.org/10.1890/0012-9658(2001)082[1205:LCAGTP]2.0.CO;2
Visconti, P. & Elkin, C., 2009, ‘Using connectivity metrics in conservation planning – When does habitat quality matter?’, Diversity and Distributions 15, 602–612. http://dx.doi.org/10.1111/j.1472-4642.2009.00564.x
Wakelin, J. & Hill, T.R., 2007, ‘The impact of land transformation on breeding Blue Swallows Hirundo atrocaerulea Sundevall, in KwaZulu-Natal, South Africa’, Journal for Nature Conservation 15(4), 245–255. http://dx.doi.org/10.1016/j.jnc.2007.05.003
Wells, K.M.S., Millsqaugh, J.J., Ryan, M.R. & Hubbard, M.W., 2008, ‘Factors affecting home range size and movements of post-fledging grassland birds’, Wilson Journal of Ornithology 120(1), 10–130. http://dx.doi.org/10.1676/06-117.1
Wethered, R. & Lawes, M., 2003, ‘Matrix effects on bird assemblages in fragmented Afromontane forests in South Africa’, Biological Conservation 114, 327–340. http://dx.doi.org/10.1016/S0006-3207(03)00052-1
Whitcomb, R.F., Robbins, C.S., Lynch, T.F., Whitcomb, B.L., Klimkiewicz, M.I.C. & Bystrak, D., 1981, ‘Effects of forest fragmentation on avifauna of the eastern deciduous forest’, in R.L. Burgess & D.M. Sharpe (eds.), Forest island dynamics in man-dominated landscapes, pp. 125–205, Springer, New York.
|